Traditional software investors have historically shied away from the world of biotech and pharma, but all of a sudden, it seems like if you’re not invested in a protein folding company, you’re actually just a loser. The appendage of .ai to the domain may have something to do with it, but the potential AI has to transform biology and drug development isn’t just a hyped pipe dream. Models like AlphaFold have become household names for scientists, and now, startups are the ones that are pushing the frontier.
Intuitively, it makes sense. Much like natural language, the nucleotides that make up RNA and DNA, the amino acids they code for that make up proteins, and even the chemicals these biomolecules interact with, are all naturally encoded in sequences. Although that is a trivialized characterization of an extremely complicated field, the ability of transformer-based architectures to understand, model, and even generate these molecules still feels almost obvious, and many are in fact exhibiting the same scaling laws we’ve seen with LLMs. Pair that with a reminder on the scale of biotech upside, and you might start feeling like a fool for not being part of the AI drug discovery party.
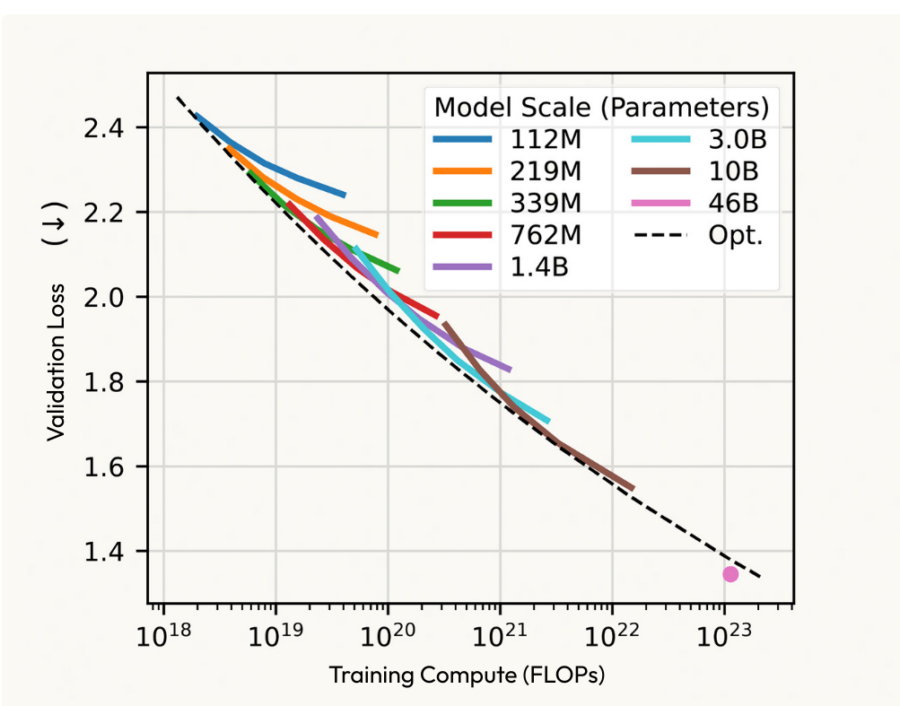
Sadly though, it’s never that simple. The industry has done a similar song and dance before.
Genome Sequencing, earlier AI waves, and numerous other bio trends have drawn in tech investors and founders, and inevitably, the complicated business models, inability to scale a software play, and uncomfortably long, risk-ridden return horizons, have spit most of us right back out. That’s not to say that there has been no place for innovative technology in drug development, but it hasn’t exactly been a layup sale. Proprietary tech or not, no one has been insulated from the fundamental economics and risk of drug trials. The biotech industry is slow, it is expensive, and it is deeply uncertain, and even the most sophisticated tech platforms have eventually capitulated to the same truth: in this space, value accrues to assets, not software.
So is everyone once again just being lured in by the same siren song? There are reasons to think things might actually be different this time, but it is not as simple as pointing to OpenAI, saying they did something no one else had, and stating that an equivalent outcome will play out here. The question to grapple with is whether AI represents a fundamentally new commercial opportunity for scientific software, or if the asset-centric economics of traditional biotech will once again dominate.
The troubled past of drug discovery tooling
No place for software
So where have things gone wrong in the past? The conclusion most of the industry seems to have landed on is that you can’t get to an outcome of scale by selling research in the form of software. You have to develop drugs. Thus far, this has largely proven true, and the industry’s only software outcomes have come from the commercial operations and data management layer (e.g., Veeva), and not from sophisticated research tools that improve the underlying science. The commonly cited example is Schrödinger, the developer of one of the most widely used computational modeling suites in the industry, who failed to get to public scale with software revenue alone and eventually developed their own drug pipeline.
This pattern played out quite clearly in the early 2000s. After the human genome was sequenced, a wave of software and data tooling startups like Celera, Gene Logic, and many others formed to capitalize on genomic data. However, the link between genes and disease turned out to be far more complex than anticipated, and translating genomic data to clinically relevant breakthroughs required years of foundational research and validation that largely came out of academia and pharma companies themselves. This meant timelines and resources that far exceeded what a startup selling horizontal software could support or monetize at the time, and many of those early startups pivoted to drug development and ultimately failed.
The platform play
Of course, that doesn’t mean there’s never been room for startups aiming to transform drug development with new technology. It’s just a bit more complicated than selling horizontal software. The commonly referred to playbook is the biotech “platform play” where rather than focusing on advancing a single, specific therapy like the traditional biotech, a startup with proprietary discovery technology commercializes on the potential to produce multiple novel assets. This technology can be either around a novel class of drugs (e.g., Moderna’s mRNA platform), or a novel way to understand existing biological mechanisms and disease pathways (e.g., Millenium did this with genomics). In both cases, in addition to internal pipelines, these biotechs will also monetize by forming major partnerships with pharma companies looking to leverage the new technology. It is what Moderna did with AstraZeneca and Roche, what Millennium did with Abbott and Bayer, and what several AI native players are establishing with Big Pharma today.
In rare cases, companies have pursued purely partnership models. Antibody platforms like Adimab and Abcellera exemplify this approach. But these partnerships aren’t SaaS licenses—they’re services agreements where the majority of the upside lies in potential royalties if a partner gets a drug to market, and as a result, the success stories are complicated and few and far between. Take Abcellera’s partnership with Eli Lilly on the COVID antibody, bamlanivimab: what looked like blockbuster revenue quickly collapsed once COVID demand faded, and without a strong internal pipeline, the company’s valuation plummeted. Ultimately, many of these partnership focused companies, including Abcellera, have come to the same conclusion that Schrodinger did: the real money is in advancing your own drugs, and unfortunately, that comes with all the unprofitable economics and a valuation that is highly dependent on potential assets rather than tech sales.
(Note: Elliot Hershberg and Patrick Malone have an excellent piece that breaks down the platform business model further).
What’s actually new about the AI wave of today
So, given the checkered past, why is everyone rushing towards the field today? The first, and most obvious answer is in fact the AI one, and while the comprehensive research synopses should be left to those with MD and PhD at the end of their names, the TLDR is that novel architectures and years of favorable data commoditization are enabling breakthroughs we have never seen before. The more nuanced argument, however, goes beyond just the tech and has more to do with the ways in which AI could shift the commercial center of gravity.
A move from proprietary assets to horizontal tooling
Past outcomes have made one thing clear: trying to sell pharma companies research in the form of discovery software is an attempt to “vendorize” an extremely complicated and inherently proprietary process. It has been a problem because all of the value in drug discovery is not in research breakthroughs but in seeing if that research actually translates to new therapies, and that process is the very bread and butter of pharma companies themselves. Thus, anyone selling the promise of new discoveries has had to bear that burden by developing their own drugs or by offering an extremely service-intensive product.
However, the capabilities of today’s state of the art biology models enable far more than just exploring unproven discoveries or drug classes. Rather they are also about (a) accelerating bottlenecks that slow down every drug pipeline across the industry, (b) automating prohibitively expensive experiments and simulations that would have previously required outsourcing to third-party vendors or investing in wet lab infrastructure, and (c) optimizing existing processes and preventing costly downstream failures.
AlphaFold 2 was the first wave of this. Its ability to model the 3D structure of proteins wasn’t unprecedented—experimental methods like X-ray crystallography had been doing it for decades, but those methods were slow and extremely resource intensive. Solving a single protein structure experimentally can take months and cost well over $100,000. AlphaFold, by contrast, could predict structures in minutes for pennies. And while X-ray crystallography remains the gold standard for atomic-resolution accuracy, AlphaFold enabled screening structural hypotheses earlier in the pipeline at dramatically higher throughput long before committing to expensive lab work.
The second order effects of AlphaFold
Of course, the obvious statement remains—AlphaFold still isn’t a business. It is an open-source project out of a research lab. When DeepMind open-sourced it, something that had long been a bottleneck and a line item on massive R&D budgets was effectively free for anyone with a laptop and an internet connection. However, it was precisely the choice to open-source the model that was crucial for the industry. By making the model freely available without the usual consulting-heavy, enterprise sales motion that dominates biopharma tooling, DeepMind forced pharma companies to adapt. There was no services team standing by to integrate the model for you. If you wanted to use AlphaFold, you had to build the in-house technical muscle to make it work, or you would get left behind. Suddenly, bio companies began investing in talent that could integrate these models directly. Although it still needs to be proved out, this shift will likely make it far easier for new AI startups to sell software into a mature buyer.
The new opportunities for AI in bio
This shift towards scientific tools with more immediate, horizontal value and the newly capable buyer base open up numerous opportunities.
Further molecular modeling
AlphaFold 2 helped collapse a single step, static protein structure prediction. But most biologically and therapeutically relevant questions are about interactions: how target proteins bind to small molecules and other proteins like drug targets, how they flex and change over time, and how their function is altered by chemical context. These are dynamic, context-dependent phenomena that require modeling tools far beyond static folding such as molecular docking, binding affinity estimation, and molecular dynamics (MD) simulations. Leading models including AlphaFold 3 (now owned by the Deepmind spinout Isomorphic) are moving in this direction, and new startups like Achira are pushing even further into simulations with models that take the crucial step of incorporating physics and quantum chemistry.
Notably, this is the same software market Schrödinger spent decades on before realizing they needed to develop their own drug pipeline to get to public scale. The problem, however, wasn’t that budgets didn’t exist—biopharma spends billions annually on these workflows—but Schrödinger’s software alone wasn’t enough to fully capture that value. Many of its core offerings faced competition from open-source tools and in-house pipelines, and as you got into particularly heavy duty simulations like MD, the bulk of real spend went towards compute rather than software licenses.
There are some signs that these patterns could be shifting. The choice to release Alphafold 3 without a commercial use license (despite criticism) might be an early sign that SOTA models will close off as economics make it harder to stomach a full open-source play at scale (sound familiar?). Additionally, as models exceed the infrastructure capacity buyers have built in house, the path of least resistance may just be to hand everything off to the models provider anyways (also sounds familiar). However, even more so than in other industries, this is a field that receives massive amounts of public and non-profit funding for open-source tooling, and that has spelled the downfall of startups in the past (e.g., there was no business for Celera in the face of the openly available Human Genome Project). Success for anyone trying to scale a pure software play in AI-driven molecular modeling will be contingent on providing step change capabilities vs. whatever is available as a commodity in open-source.
Downstream prediction & testing
Of course, molecular modeling is far from the only application where AI is making meaningful inroads. An unfortunate reality remains that around ninety percent of clinical-stage molecules still fail, and most of these failures are a result of efficacy and safety issues that do not tend to be entirely predictable from molecular properties like binding or distribution. Take toxicity, one of the most expensive and failure-prone choke points in drug development. Preclinical lab and animal safety testing eats up billions in spend each year, but the accuracy of these tests remains disturbingly low. As a result, toxicity remains a leading cause of clinical failures and drug recalls, and billions in sunk R&D costs get burned as a result.
The market is one that reaches far beyond pharma—the chemicals, cosmetics, and agriculture industries are all massive spenders here. And aside from eye-popping spend, what makes it one of the most attractive opportunities for disruption across all these sectors, is the fact that buyers aren’t looking for some sort of proprietary insight, they just want a simple go/no-go signal. As a result, most of the work just ends up getting outsourced to whatever external vendor provides the most accurate and affordable test.
There is still the fairly obvious statement that software TAM ≠ wet lab spend, but nonetheless, the models looking to disrupt this market do not need to be a one-to-one replacement for animal and in-vitro tests. Achieving that requires substantial regulatory and cultural change, but there are large businesses like Certara in adjacent biosimulation markets that have shown that there is a meaningful market even just for being complimentary to traditional methods. Moreover, with (a) leading startups like Axiom showing that AI models may actually exceed experimental capabilities altogether and (b) the FDA announcing plans to phase out animal testing, the possibility of actually replacing wet lab TAM may in fact be closer than we think. The biggest risk is going to be tech execution—these models are fundamentally different from protein modeling, and the translation of the research to truly commercially viable tests in practice is still to be proven.
Design optimization & de novo design
Now, this is where many of the headline makers and accolades have come from. Here, the focus shifts from modeling known targets or drug candidates within existing libraries to generating entirely new molecules. That includes using protein models to design novel biologic therapies, as well as generative chemistry to discover new small molecule leads. Both present a massive opportunity to shift drug discovery to a much more deliberate and iterative design process, and efforts range all the way from design optimization—refining known leads to improve stability, safety, manufacturability, etc., all the way to de novo design, where models generate completely novel molecules from scratch based on a biological “prompt.”
The discerning reader would be quick to point out, however, that this is very much not a move away from technology that focuses on proprietary assets. Like many of the platform plays that have come before, the applications for these models are predominantly around surfacing novel, revenue generating molecules and insights, and that fact shows up in the wide spread of business models in this space.
For the billion and near billion dollar fundraisers like Xaira and Isomorphic, all signs point towards full-on biotechs with internal pipelines. Other players like Cradle are gunning for a pure software play that integrates into the existing workflows and bets on a newly matured buyer base with the talent and infrastructure ready to adopt out-of-the box tooling. And somewhat down the middle, players like Latent Labs and Profluent are taking a more partnership-based approach.
Regardless of the business model, however, an additional and clear “what’s different this time” is also just AI. At the risk of stating the obvious, the novelty and rate of progress of this research is not like anything that has come before it, and where that has been most clearly demonstrated is not only in protein modeling, but generative capabilities writ large. Pharma companies, however, simply do not have the AI research talent to build foundation models themselves. Thus, the onus will be on the startups, and there will likely be many ways up the same mountain.
Final thoughts
Ultimately, there are massive opportunities for investors and builders across this entire field. The spaces outlined above only begin to scratch the surface of what is happening, and there are even greater moonshot ideas coming out of companies like Bioptimus and Somite, research labs like the Arc Institute, and various players targeting issues further downstream within the clinical trial process. These are all worth entire blog posts of their own to unpack. It is a field that is incredibly rich and incredibly deep.
As an AI and software-focused investor, some of the most exciting opportunities will lie in utilizing AI to disrupt massive line items that are long overdue for software disruption and still far from any sort of AI commoditization. Here, the risk is not pharmacological or clinical. It is technical. And technical risk is what AI and software investors are built to underwrite.
Having said that, what is happening in protein design and generation is hard to ignore. The upside is staggering, and the research breakthroughs and the scaling laws being demonstrated make a very compelling case for joining the party. Even though AI is changing things substantially, however, these are still predominantly asset-based bets. It is pretty likely that someone entering it from the outside with software-level risk tolerance and biotech-level return expectations will end up disappointed, but for those that have the stomach, the upside could be nearly uncapped.